Scaling Generative AI has emerged as one of the most transformative technologies of our time, reshaping industries, redefining customer experiences, and unlocking unprecedented opportunities for innovation. By leveraging advanced algorithms, generative AI enables machines to produce creative outputs like text, images, videos, and even product designs, which were traditionally the domain of human ingenuity. In 2025, this technology stands at a critical inflection point, with organizations eager to scale its capabilities and integrate it into their core operations.
For data leaders, the stakes are higher than ever. While generative AI offers immense potential, the challenges of implementation, scalability, and ethical considerations cannot be ignored. Questions about data quality, computational costs, and responsible usage loom large. Yet, those who can successfully navigate these complexities have the chance to drive unparalleled growth, improve operational efficiency, and gain a competitive edge in their industries.
This blog serves as a roadmap for data leaders, outlining strategies to scale generative AI responsibly and effectively. From fostering collaboration across teams to investing in robust technology infrastructure and talent, we’ll explore the key components of a successful generative AI strategy. By the end, you’ll be equipped with actionable insights to unlock the full value of generative AI in 2025 and beyond.
The Generative AI Landscape in 2025
Generative AI technologies like large language models (LLMs), generative adversarial networks (GANs), and diffusion models are transforming the way businesses operate. These technologies are now widely used in applications ranging from automating customer support to creating hyper-personalized marketing content. In the healthcare sector, generative AI is enabling breakthroughs in drug discovery, while retail is leveraging AI to predict consumer behavior and optimize supply chains.
However, the journey to scaling generative AI is fraught with challenges. Data bottlenecks, computational costs, and ethical concerns remain significant barriers. Additionally, many organizations struggle to move beyond isolated AI experiments to full-scale implementation. Despite these hurdles, those who succeed in scaling generative AI stand to gain a competitive edge, reaping benefits such as enhanced efficiency, increased revenue, and improved customer satisfaction.
The Role of Data Leaders in Driving Generative AI Success
Data leaders are the architects of AI transformation within their organizations. Their role is multifaceted, requiring a blend of technical expertise, strategic vision, and leadership skills. Here are three critical ways data leaders can drive generative AI success:
- Strategic Vision: Generative AI should be integrated into the broader business strategy, aligning with goals such as revenue growth, cost reduction, and customer satisfaction. Data leaders must identify high-impact use cases that can deliver measurable value.
- Cross-Functional Collaboration: The success of generative AI initiatives often depends on collaboration across departments. Data leaders should bridge the gap between technical teams and business stakeholders, ensuring alignment on objectives and outcomes.
- Governance and Ethics: As stewards of AI adoption, data leaders must establish governance frameworks to manage risks, ensure compliance, and promote ethical AI practices.
By adopting a holistic approach, data leaders can turn generative AI from a buzzword into a strategic asset.
Critical Components for Scaling Generative AI
Scaling generative AI requires a focus on three critical areas: data, technology, and talent.
1. Data Strategy
A strong data strategy is the backbone of successful generative AI initiatives. Key considerations include:
- Data Quality and Diversity: Generative AI models perform best when trained on high-quality, diverse datasets. Organizations must invest in data cleaning and augmentation processes to ensure accuracy and reliability.
- Ethical Data Practices: Addressing biases in training data is crucial for producing fair and equitable AI outputs. Data leaders should implement measures to identify and mitigate potential biases.
- Data Governance: Robust governance frameworks are essential for ensuring data security, compliance, and ethical use.
2. Technology Infrastructure
The right technology infrastructure is critical for scaling generative AI. This includes:
- Scalability and Flexibility: Cloud computing platforms offer the scalability needed to handle resource-intensive AI workloads. Edge computing can also be leveraged for real-time processing.
- AI Platforms and Toolkits: Pre-built AI platforms, such as OpenAI and Hugging Face, can accelerate deployment by providing ready-to-use models and APIs. Organizations can also develop custom solutions tailored to their unique needs.
- Cost Management: Generative AI requires significant computational power, which can be expensive. Adopting efficient algorithms and optimizing resource usage can help reduce costs.
3. Talent Development
To scale generative AI effectively, organizations must invest in their workforce. This involves:
- Upskilling and Reskilling: Training programs should focus on equipping employees with the skills needed to develop, deploy, and manage generative AI systems.
- Interdisciplinary Collaboration: Generative AI often requires input from data scientists, engineers, and domain experts. Fostering collaboration across teams can lead to more innovative solutions.
- AI Literacy: Non-technical staff should also be educated on the basics of generative AI to facilitate better decision-making and adoption.
Overcoming Barriers to Scaling Generative AI
While the potential of generative AI is immense, several barriers must be addressed to realize its full value:
- Data Privacy and Compliance: With growing concerns about data security, organizations must ensure their AI systems comply with regulations like GDPR and CCPA. Transparent data handling practices can help build trust with stakeholders.
- Cost Optimization: The computational demands of generative AI can strain budgets. Organizations should explore cost-effective solutions, such as leveraging open-source tools or partnering with cloud service providers.
- From Experimentation to Implementation: Many organizations struggle to scale AI beyond pilot projects. This requires a shift in mindset, with a focus on building scalable solutions, setting clear KPIs, and ensuring stakeholder alignment.
Industry-Specific Use Cases for Generative AI
Generative AI is delivering value across various industries. Here are a few notable examples:
- Healthcare: AI-powered drug discovery, personalized treatment plans, and medical imaging analysis.
- Retail: AI-driven inventory management, personalized recommendations, and dynamic pricing strategies.
- Finance: Fraud detection, risk assessment, and AI-generated financial reports.
- Media and Entertainment: Automated content generation, video editing, and localization of multimedia assets.
These use cases highlight the versatility of generative AI and its potential to drive innovation across sectors.
Setting a Visionary Agenda for 2025
To scale generative AI successfully, data leaders must set a clear agenda. This includes:
- Developing a Roadmap: Define specific goals, timelines, and milestones for AI initiatives.
- Measuring Success: Use KPIs such as ROI, model performance, and user adoption to track progress.
- Fostering Partnerships: Collaborate with AI vendors, research institutions, and startups to stay ahead of technological advancements.
The Ethical Imperative: Responsible AI at Scale
As generative AI becomes more pervasive, ethical considerations must remain a top priority. Data leaders should focus on:
- Transparency: Ensure AI systems are explainable and interpretable.
- Fairness: Address biases in AI models to promote inclusivity.
- Accountability: Establish mechanisms to monitor and address potential risks associated with AI deployment.
Organizations can build trust with stakeholders and ensure sustainable AI adoption by prioritizing ethical practices.
Conclusion: The Road Ahead for Data Leaders
Generative AI holds immense promise as a catalyst for innovation and growth, but realizing its full potential requires more than cutting-edge technology. For data leaders, 2025 represents an opportunity to scale AI responsibly, align it with strategic goals, and address the ethical and operational challenges that come with it.
By building robust data pipelines, investing in scalable technology, and fostering interdisciplinary collaboration, organizations can create AI systems that deliver measurable value. Equally important is a commitment to responsible AI practices, ensuring fairness, transparency, and accountability in every application.As the generative AI landscape continues to evolve, staying informed and agile will be key. Data leaders who embrace innovation with a balanced approach will not only unlock significant business value but also pave the way for sustainable, ethical AI adoption. Discover more insights about generative AI and its impact on industries at Technado.
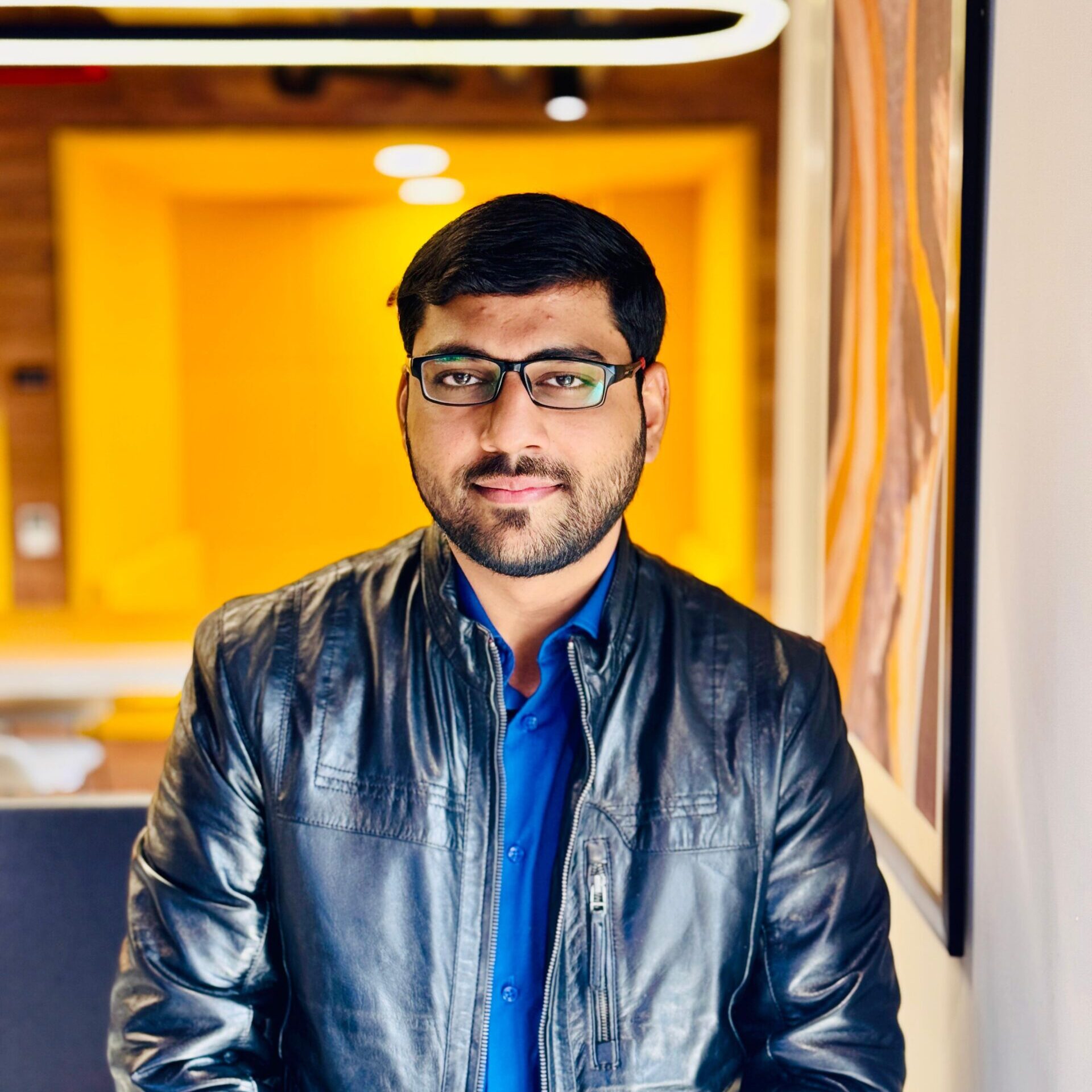
Jahanzaib is a Content Contributor at Technado, specializing in cybersecurity. With expertise in identifying vulnerabilities and developing robust solutions, he delivers valuable insights into securing the digital landscape.