In the relentless race of artificial intelligence (AI) development, hardware performance is often celebrated as the ultimate benchmark of progress. When Nvidia recently announced that its latest GPUs are the fastest for DeepSeek AI, the news rippled across the tech world. After all, Nvidia has long been a dominant force in high-performance computing, consistently delivering powerful hardware that fuels the AI revolution.
But while the claim of “fastest GPUs” is undoubtedly impressive, it also begs a crucial question: Is speed really the defining factor in AI advancement? AI is more than just a computational arms race—it’s an ecosystem where software, algorithms, efficiency, and scalability play equally vital roles. By focusing solely on speed, Nvidia might be missing the bigger picture.
Let’s break down why the real success of AI development isn’t just about processing power but about a more holistic approach that blends efficiency, accessibility, and real-world applicability.
The Hype Around Nvidia’s Latest GPUs: Marketing vs. Reality
Nvidia has a long history of setting new performance benchmarks in AI and deep learning. The company’s latest generation of GPUs is packed with cutting-edge architecture, enhanced memory capabilities, and optimized AI accelerators designed to process vast amounts of data at unprecedented speeds.
But what does “fastest for DeepSeek AI” actually mean?
On the surface, Nvidia’s claim suggests that their new GPUs provide superior processing speeds for DeepSeek AI’s workloads, whether that’s training large-scale neural networks or running inference at lightning-fast rates. This kind of marketing messaging taps into the growing demand for AI acceleration, particularly as enterprises and researchers push the boundaries of generative AI, autonomous systems, and large language models.
However, the focus on speed alone can be misleading. AI success isn’t just about who can process data the fastest—it’s about how efficiently and effectively that processing power translates into real-world impact.
What Is DeepSeek AI, and Why Does Speed Matter?
DeepSeek AI represents a broader category of AI-driven search and deep learning models designed to extract insights from vast datasets. These models require substantial computing power to train and deploy, making GPU speed an important factor.
For example, when training a large AI model, faster GPUs can significantly reduce the time required to complete computations. This can lead to quicker iterations, faster research cycles, and improved responsiveness in AI-driven applications.
However, while speed is beneficial, it is not the only factor that determines AI success. In many cases, the ability to efficiently scale, optimize software frameworks, and reduce energy consumption can be just as—if not more—important than raw processing power.
Why Speed Alone Isn’t Enough in AI Development
While Nvidia’s claim of superior speed is impressive from a technical standpoint, the reality is that AI advancement depends on more than just GPU benchmarks. Let’s explore some of the overlooked aspects that play a crucial role in AI progress.
1. Energy Efficiency and Sustainability
One of the biggest challenges in AI is the increasing demand for energy. High-performance GPUs consume vast amounts of electricity, contributing to higher operational costs and environmental concerns.
For large-scale AI operations—such as training massive language models—energy efficiency is often a greater concern than raw processing speed. Companies and researchers are actively exploring more sustainable solutions, including low-power AI chips and optimized software that reduces computational waste.
If Nvidia’s new GPUs offer record-breaking speeds but come at the cost of higher power consumption, the long-term benefits may be questionable. Efficiency should be just as much a priority as performance.
2. Software Optimization and AI Algorithms
AI performance doesn’t just depend on hardware; software plays an equally important role. Advances in AI algorithms, machine learning frameworks, and data management techniques can lead to significant performance gains without requiring hardware upgrades.
For instance:
- New AI model architectures like transformers and diffusion models have made AI systems more efficient.
- Software libraries like TensorFlow and PyTorch continuously introduce optimizations that reduce the need for brute-force computing power.
- Techniques like model pruning, quantization, and knowledge distillation allow AI models to run faster on existing hardware without sacrificing accuracy.
In many cases, software optimization can outperform raw hardware improvements, making it crucial to consider AI as a complete ecosystem rather than just a hardware-dependent field.
3. Scalability and Cost-Effectiveness
AI systems need to be scalable—both in terms of hardware and cost. Organizations working with AI aren’t just looking for the fastest GPUs; they need solutions that are economically viable and capable of scaling up to handle increasing workloads.
A GPU that boasts the highest performance might not be the best choice if it comes with excessive costs, compatibility issues, or limited flexibility. Cloud-based AI services, edge computing, and distributed AI architectures are becoming increasingly popular alternatives to high-end, power-hungry GPUs.
4. Real-World AI Applications vs. Benchmark Performance
Benchmark scores provide a useful way to compare hardware, but they don’t always translate to real-world performance. AI applications span a wide range of industries, including healthcare, finance, and autonomous systems, each with unique demands.
For example:
- In medical AI, interpretability and reliability matter more than sheer speed.
- In autonomous vehicles, AI needs to process data in real time but must also prioritize safety and robustness.
- In enterprise AI, integration with existing infrastructure is often a bigger challenge than computation speed.
A GPU optimized for DeepSeek AI might excel in lab tests but could struggle in practical deployment if it doesn’t consider these real-world constraints.
Beyond Hardware: The Future of AI Innovation
Rather than viewing AI advancement as a simple matter of “who has the fastest GPU,” it’s more productive to focus on a balanced approach that considers the following:
1. Hardware-Software Co-Optimization
Instead of relying solely on GPU speed improvements, AI companies should invest in developing smarter software that can extract maximum efficiency from available hardware. Innovations in AI algorithms and programming techniques can often deliver performance gains that rival new hardware releases.
2. Energy-Conscious AI Development
With AI computing demands increasing exponentially, energy efficiency will become a defining factor. Future AI development must focus on balancing power consumption with computational speed to ensure sustainable progress.
3. Cloud and Edge AI
Cloud-based AI platforms and edge computing are gaining traction as more efficient alternatives to high-end GPUs. By offloading AI workloads to the cloud or running AI models closer to data sources (e.g., IoT devices), organizations can achieve high performance without relying on ultra-powerful local hardware.
4. AI Democratization and Accessibility
The true impact of AI isn’t just in how fast it runs but in how widely it can be deployed. Making AI tools accessible to startups, researchers, and enterprises of all sizes is just as important as raw speed. Lowering hardware costs, improving software interoperability, and providing open-source AI solutions can drive innovation across industries.
Final Thoughts: Speed Matters, but It’s Not the Whole Story
Nvidia’s latest GPUs are undoubtedly a technological marvel, boasting impressive speed and capabilities. But the claim that they are the “fastest for DeepSeek AI” reflects a narrow view of AI development—one that prioritizes raw performance over a more holistic understanding of what truly drives AI progress.
Speed is valuable, but it is just one piece of the puzzle. AI’s future will be shaped by efficiency, scalability, cost-effectiveness, and real-world applicability. Companies, researchers, and developers must look beyond performance benchmarks and focus on building AI systems that are not only powerful but also practical, sustainable, and accessible.
At the end of the day, the AI race isn’t about who has the fastest hardware—it’s about who can deliver the most meaningful and impactful innovations.
What do you think? Should AI hardware manufacturers focus more on speed, or should they take a broader approach? Let us know in the comments!
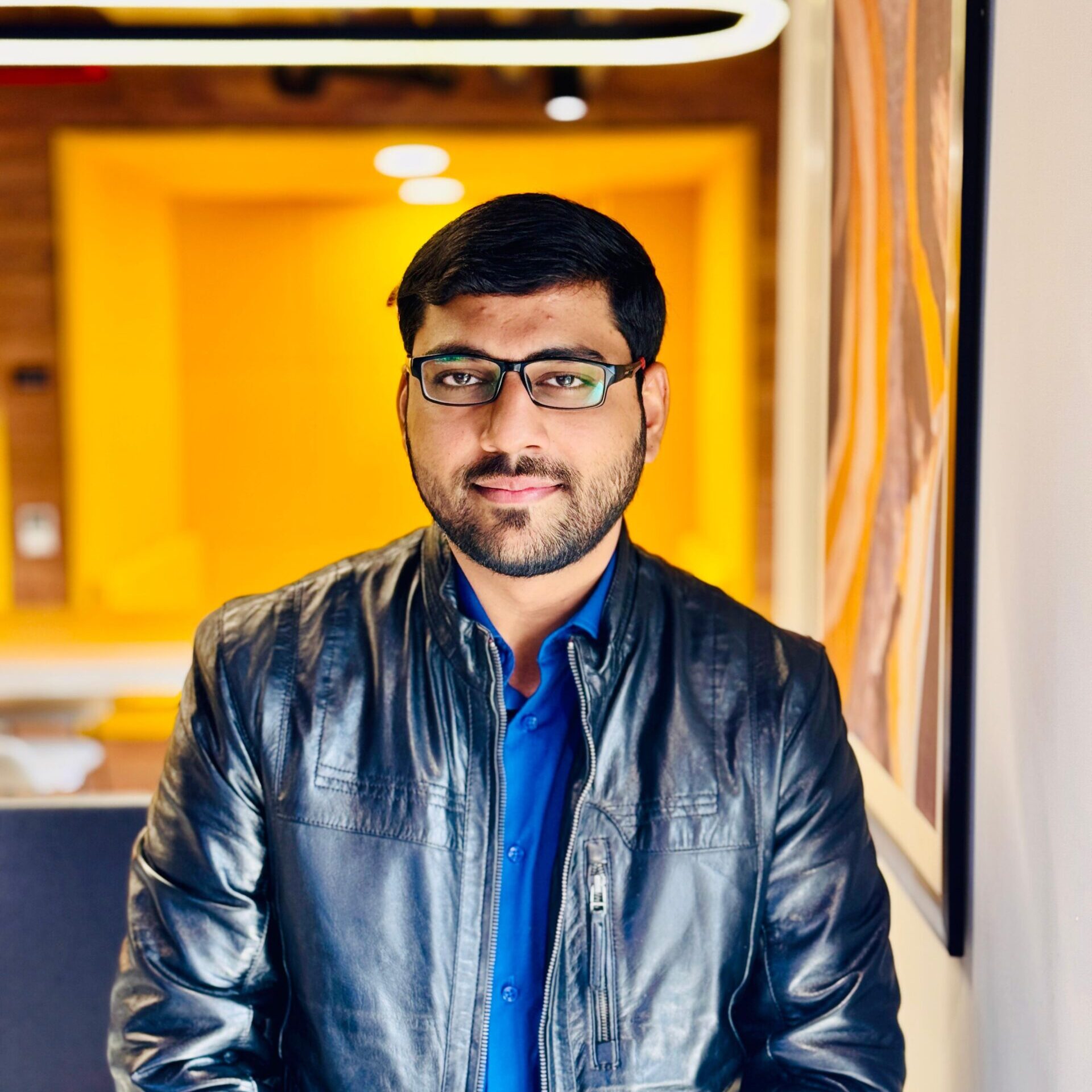
Jahanzaib is a Content Contributor at Technado, specializing in cybersecurity. With expertise in identifying vulnerabilities and developing robust solutions, he delivers valuable insights into securing the digital landscape.