Generative AI has become one of the most discussed and revolutionary technologies in recent years. With its ability to create text, images, music, and even software code, it’s clear that generative AI has the potential to change the way we work, create, and innovate. From automated content creation to helping artists generate novel works of art, generative AI promises to open new doors in virtually every sector. However, despite the growing excitement and media attention, generative AI still has a long way to go before it can prove its real-world usefulness in a meaningful, widespread way.
The Rise of Generative AI: Unlocking New Possibilities
Generative AI refers to a class of machine learning models that have the ability to generate new data based on existing patterns. By learning from large datasets, these models can produce human-like text, realistic images, and even create music or video. Some of the most notable examples of generative AI include GPT-4, DALL-E, and other advanced AI models capable of generating text and images, respectively.
Businesses have quickly adopted generative AI to assist with everything from creating product descriptions and marketing content to streamlining customer support and even designing new products. In creative industries, it has sparked a new wave of innovation, with AI models being used to generate art, write stories, and compose music.
While generative AI has proven effective in many cases, its potential is far from fully realized. The technology’s rapid development has raised high expectations, but there are still significant hurdles that prevent it from being truly useful in real-world applications. For generative AI to live up to its promise, it needs to overcome these challenges and prove its value in practical, impactful ways.
The Challenges of Proving Generative AI’s Usefulness
Generative AI holds great promise, but its implementation in real-world scenarios has yet to be smooth. Below are some of the key challenges that still need to be addressed for it to fully demonstrate its usefulness:
1. Inconsistent Quality and Accuracy of Outputs
One of the most significant hurdles facing generative AI is the inconsistency in the quality and accuracy of its outputs. While the AI models can generate text, images, or other forms of content that appear impressive at first glance, they often struggle to maintain high quality over longer stretches or more complex tasks. In text generation, for example, models like GPT-4 may produce coherent and relevant content in some contexts, but they are also prone to errors, inconsistencies, and nonsensical output in others.
In high-stakes industries such as healthcare, law, or finance, where precision and accuracy are critical, the potential for generative AI to make mistakes is a significant concern. Even minor errors in content generation can have major consequences. For instance, an AI-generated report with factual inaccuracies could lead to poor decision-making, affecting businesses, individuals, or entire industries.
To prove its usefulness, generative AI must produce outputs that are reliable, accurate, and consistent, especially in professional and high-risk environments.
2. Ethical and Legal Concerns
With the rise of generative AI, many ethical and legal concerns have surfaced. One major issue revolves around authorship and ownership. If an AI model generates a piece of content, such as an article, artwork, or song, who owns the rights to that work? This question has important implications for intellectual property, copyright, and fair use laws.
Moreover, generative AI is known to perpetuate biases present in the data it is trained on. Since AI models learn from large datasets, they may inadvertently reproduce stereotypes or biased viewpoints if those biases are embedded in the data. This can lead to harmful outcomes, especially in sensitive areas like hiring practices, legal judgments, or social media content moderation.
In addition, generative AI can be used to create realistic but fake content—such as deepfake videos or AI-generated news articles—which could be used to spread misinformation or manipulate public opinion. The ethical dilemma here is not just about the content itself, but also about who is responsible for the content’s creation and dissemination.
To address these concerns, businesses and governments must establish strict guidelines for the ethical use of generative AI. Clear policies around ownership, accountability, and the mitigation of bias are essential to ensure that AI can be deployed in a responsible and trustworthy manner.
3. Dependency on High-Quality Data
Generative AI’s performance is heavily dependent on the quality of the data it’s trained on. AI models learn patterns from data, and if the data is incomplete, outdated, or biased, the resulting outputs will reflect those same issues. For example, if an AI model is trained on biased or one-sided data, it may generate outputs that are prejudiced or narrow in scope.
In real-world applications, AI is often required to work with diverse datasets that reflect a broad range of perspectives and contexts. Whether it’s training a chatbot to assist customers from various backgrounds or generating content for different cultural contexts, data quality becomes a key determinant of AI’s effectiveness.
Furthermore, the issue of “data privacy” comes into play. In some cases, the data required to train AI models may contain sensitive or personal information, which raises questions about data security and consent. AI systems must be trained on ethically sourced data, and strict privacy protocols must be in place to ensure that the use of generative AI does not compromise individuals’ privacy or lead to data misuse.
4. Real-World Adoption and Integration
Despite the hype surrounding generative AI, its integration into real-world applications is still in its early stages. While businesses have started experimenting with AI in specific areas like content generation or automation, full-scale adoption remains limited. Many industries are still uncertain about how best to integrate AI into their existing workflows, and AI is often used as a complementary tool rather than as a primary solution.
For example, AI tools may assist in creating social media posts, writing reports, or drafting marketing content, but human input is still required to ensure that the final output aligns with the brand tone, quality standards, and specific business goals. This hybrid approach—where AI assists but does not fully replace human input—has proven to be the most effective in many cases.
Furthermore, the cost of implementing generative AI technologies can be prohibitive for smaller businesses, which limits their ability to harness the technology’s potential. While some organizations have the resources to invest in sophisticated AI models, others may struggle to keep up with the rapid pace of technological change.
5. AI Creativity vs. Human Creativity
Generative AI has demonstrated its ability to produce creative works such as poems, music, and visual art. However, there remains a debate about whether AI can truly be creative or if it is simply mimicking creativity by producing outputs based on learned patterns.
AI can replicate artistic styles or generate works that resemble human creativity, but it often lacks the spark of true innovation. Human creativity is driven by personal experiences, emotions, and unique perspectives, which AI cannot replicate. While AI can be used as a tool to assist in creative processes, it cannot replace the depth and originality that comes from human imagination and intuition.
In industries like art, music, and literature, where originality is highly valued, generative AI’s role is likely to remain as a tool for augmentation rather than a replacement for human creators.
The Path Forward: Proving Generative AI’s Value
Despite these challenges, generative AI holds immense potential, and with the right advancements, it can become a truly useful tool across various sectors. Here’s how generative AI can evolve and prove its value in the coming years:
1. Improved Accuracy and Consistency
To prove its usefulness, generative AI must improve in accuracy and consistency. Developers must continue to refine the algorithms and expand the datasets used for training. Better training methods, more diverse data, and improved validation processes can help mitigate the issues of inconsistency and factual inaccuracy. AI should also become more adaptable, allowing users to provide feedback to fine-tune outputs in real-time.
2. Clear Ethical Guidelines and Regulation
As generative AI becomes more prevalent, establishing robust ethical guidelines will be essential. Industry-wide standards for transparency, accountability, and bias mitigation should be put in place to ensure responsible use. Governments and regulatory bodies will need to play an active role in monitoring and enforcing these standards, ensuring that generative AI operates within ethical and legal boundaries.
3. AI-Human Collaboration for Enhanced Creativity
The future of generative AI lies in collaboration with humans, rather than in replacing them. AI should be seen as a tool that amplifies human creativity and efficiency, rather than a substitute for human expertise. By combining human intuition and creativity with AI’s ability to process vast amounts of data quickly, businesses can unlock new levels of innovation and productivity.
4. Widespread Adoption and Integration
For generative AI to become truly useful, businesses must continue to experiment and integrate it into their core operations. As more companies see the value in AI tools, the technology will become more refined and accessible. Collaboration between tech developers and businesses will help identify the most effective ways to leverage AI, whether it’s for automating repetitive tasks, generating content, or driving innovation in product development.
Conclusion: A Work in Progress with Enormous Potential
Generative AI has made great strides in recent years, demonstrating its potential to transform industries and open up new possibilities. However, its usefulness in the real world still needs to be proven. As we navigate the challenges of quality, ethics, data, and adoption, generative AI will evolve. Through continued advancements, careful regulation, and collaboration between humans and AI, this technology can fulfill its potential and become an indispensable tool in business, creativity, and beyond. The road ahead is long, but with the right investments and a focus on responsible development, the future of generative AI is bright.
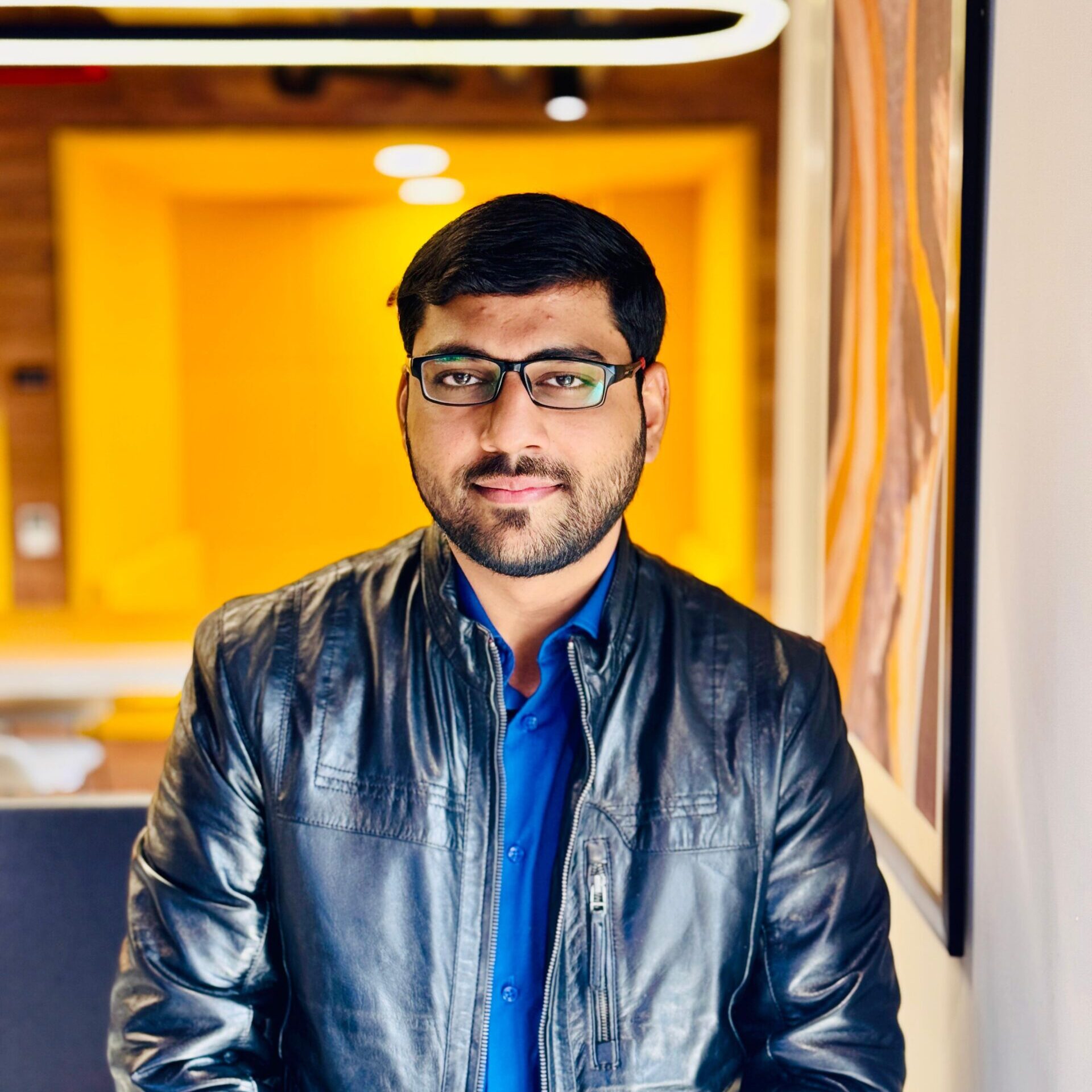
Jahanzaib is a Content Contributor at Technado, specializing in cybersecurity. With expertise in identifying vulnerabilities and developing robust solutions, he delivers valuable insights into securing the digital landscape.